Unlocking the Future: The Importance of Training Data for Self-Driving Cars
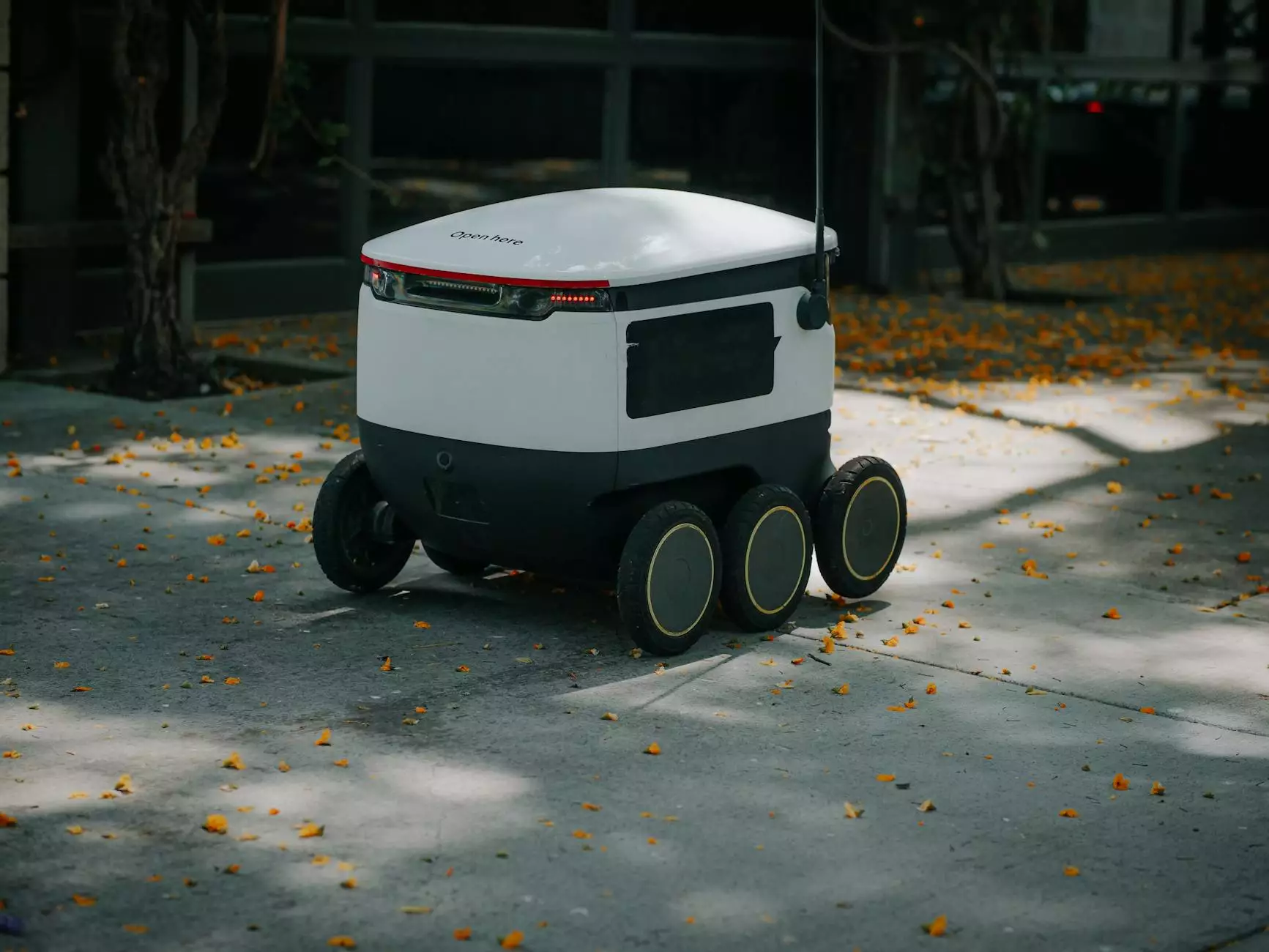
In an era where technology is advancing at an unprecedented pace, self-driving cars stand out as one of the most revolutionary developments in the automotive industry. Central to the success and safety of these autonomous vehicles is a crucial element: training data for self-driving cars. This article delves deeply into the significance of high-quality training data, how it drives innovation in the industry, and the implications for the future of transportation.
What is Training Data for Self-Driving Cars?
The term training data for self-driving cars refers to the vast amounts of information collected and used to train machine learning models, enabling vehicles to navigate safely and efficiently. This data encompasses various inputs, including:
- Visual Data: Images and videos from cameras positioned around the vehicle.
- Lidar and Radar Data: 3D representations of the environment created by Lidar sensors and distance measurement from radar systems.
- GPS Information: Location data that helps cars understand their physical location on the map.
- Traffic and Environmental Data: Information about road conditions, traffic signals, pedestrian activity, and weather impacts.
Why Is Training Data Essential?
Training data provides the foundation for machine learning algorithms, allowing them to recognize patterns, make predictions, and adapt to changing environments. The necessity of this data can be understood through the following points:
1. Safety and Reliability
When it comes to self-driving technology, safety is the foremost priority. Training data is critical in ensuring these vehicles can handle a multitude of scenarios. Large, diverse datasets allow the algorithms to learn from previous incidents, enhancing their ability to make safe decisions in real-time.
2. Continuous Learning
Self-driving cars operate in a rapidly changing environment. As traffic patterns and road conditions evolve, the data used for training allows these vehicles to continuously learn and improve. This ongoing process reduces the risk of accidents caused by outdated algorithms, ensuring that vehicles adapt to the latest trends and challenges.
3. Enhancing User Experience
A well-trained self-driving car not only prioritizes safety but also enhances user experience. By analyzing training data, these vehicles can learn routes that minimize travel time while maximizing comfort, leading to a smoother ride for passengers.
The Process of Creating Quality Training Data
The creation of effective training data is a meticulous process that involves several key steps:
Data Collection
To build a comprehensive dataset, self-driving technology companies deploy a fleet of vehicles equipped with sensors and cameras. These vehicles collect vast amounts of data while driving in various conditions, covering urban streets, highways, rural roads, and special situations such as construction zones and adverse weather conditions.
Data Annotation
Once the data is collected, it must be annotated accurately. This process involves labeling the data to help the algorithms understand context. For example, annotators may categorize objects like pedestrians, other vehicles, cyclists, traffic lights, and road signs within images or videos.
Data Validation
After annotation, the training data undergoes rigorous validation. This step ensures that the labels are correct and that the quality of data meets the required standards. Only validated datasets are used for training machine learning models.
Challenges in Creating Quality Training Data
Despite its importance, creating high-quality training data for self-driving cars comes with its challenges:
1. Diverse Scenarios
Self-driving cars need to be trained on a wide variety of scenarios, which means the data must reflect diverse environments, weather conditions, and human behavior. Achieving this diversity can be time-consuming and resource-intensive.
2. Data Privacy Concerns
Collecting data in public spaces raises questions about privacy and ethical use of information. Companies must navigate legal regulations and public perception while gathering data.
3. Real-Time Adaptation
Handling real-time data and continuously feeding new information into the training models pose significant technical challenges. Ensuring that the models adapt dynamically without compromising safety remains a top priority for developers.
The Future of Training Data and Self-Driving Cars
As technology progresses, the future of training data for self-driving cars looks promising. Key trends include:
1. Use of Synthetic Data
Synthetic data generation is gaining traction in the industry. Engineers can create virtual environments to simulate various driving conditions, helping to augment real-world data without the constraints of time and geography.
2. Collaborative Data Sharing
Collaboration among companies may lead to richer datasets for training. Shared data can enhance the overall safety and efficiency of self-driving technology by allowing algorithms to learn from a broader spectrum of experiences.
3. Enhanced AI Capabilities
Machine learning and AI techniques will continue to evolve, leading to smarter algorithms that require less data to learn effectively. This will streamline the training process, making it easier to deploy self-driving technology across different vehicles.
Conclusion: The Road Ahead
In conclusion, training data for self-driving cars is not merely a technical requirement but a vital component that shapes the future of transportation. As we move toward an autonomous era, the focus on collecting, validating, and utilizing high-quality data will be paramount. It affects not only the safety and efficiency of self-driving cars but also the overall adoption of this transformative technology.
For businesses in the Home Services and Keys & Locksmiths sectors, the implications are profound. Increased reliance on technology will demand that they keep pace with innovations like self-driving delivery vehicles, automated locksmith services, and beyond. Embracing change and understanding the importance of training data will enable these businesses to thrive in a rapidly evolving landscape.
As we witness the convergence of technology and traditional industries, it’s clear that the future holds limitless possibilities. By prioritizing quality training data, businesses can not only contribute to the safety of autonomous driving but also unlock new growth opportunities in their sectors. The journey towards a fully autonomous driving future is exciting, and the importance of training data will remain a key driver in this transformative journey.
training data for self driving cars